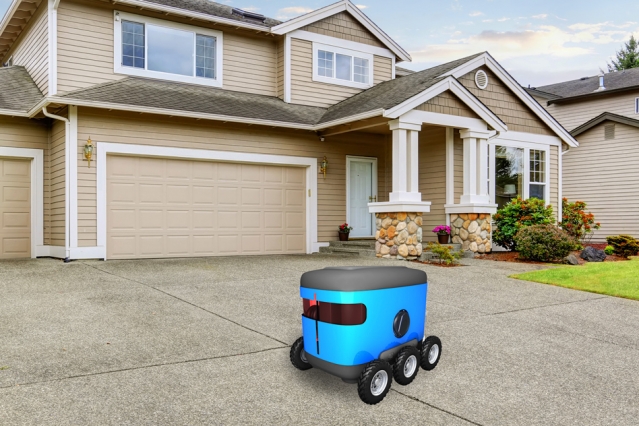
Last-mile delivery robots could use an MIT algorithm to find the front door, using environmental clues. | Credit: MIT
In the not too distant future, last-mile delivery robots may be to drop your takeout order, package, or meal-kit subscription at your doorstep – if they can find the door.
Standard approaches for robotic navigation involve mapping an area ahead of time, then using algorithms to guide a robot toward a specific goal or GPS coordinate on the map. While this approach might make sense for exploring specific environments, such as the layout of a particular building or planned obstacle course, it can become unwieldy in the context of last-mile delivery robots.
Imagine, for instance, having to map in advance every single neighborhood within a robot’s delivery zone, including the configuration of each house within that neighborhood along with the specific coordinates of each house’s front door. Such a task can be difficult to scale to an entire city, particularly as the exteriors of houses often change with the seasons. Mapping every single house could also run into issues of security and privacy.
Now MIT engineers have developed a navigation method that doesn’t require mapping an area in advance. Instead, their approach enables a robot to use clues in its environment to plan out a route to its destination, which can be described in general semantic terms, such as “front door” or “garage,” rather than as coordinates on a map. For example, if a robot is instructed to deliver a package to someone’s front door, it might start on the road and see a driveway, which it has been trained to recognize as likely to lead toward a sidewalk, which in turn is likely to lead to the front door.
Related: Delivery tests combine autonomous vehicles, bipedal robots
The new technique can greatly reduce the time last-mile delivery robots spend exploring a property before identifying its target, and it doesn’t rely on maps of specific residences.
“We wouldn’t want to have to make a map of every building that we’d need to visit,” says Michael Everett, a graduate student in MIT’s Department of Mechanical Engineering. “With this technique, we hope to drop a robot at the end of any driveway and have it find a door.”
Everett presented the group’s results at the International Conference on Intelligent Robots and Systems. The paper, which is co-authored by Jonathan How, professor of aeronautics and astronautics at MIT, and Justin Miller of the Ford Motor Company, is a finalist for “Best Paper for Cognitive Robots.”
“A sense of what things are”
In recent years, researchers have worked on introducing natural, semantic language to robotic systems, training robots to recognize objects by their semantic labels, so they can visually process a door as a door, for example, and not simply as a solid, rectangular obstacle.
“Now we have an ability to give robots a sense of what things are, in real-time,” Everett says.
Everett, How, and Miller are using similar semantic techniques as a springboard for their new navigation approach, which leverages pre-existing algorithms that extract features from visual data to generate a new map of the same scene, represented as semantic clues, or context.
In their case, the researchers used an algorithm to build up a map of the environment as the robot moved around, using the semantic labels of each object and a depth image. This algorithm is called semantic SLAM (Simultaneous Localization and Mapping).
While other semantic algorithms have enabled robots to recognize and map objects in their environment for what they are, they haven’t allowed a robot to make decisions in the moment while navigating a new environment, on the most efficient path to take to a semantic destination such as a “front door.”
“Before, exploring was just, plop a robot down and say ‘go,’ and it will move around and eventually get there, but it will be slow,” How says.
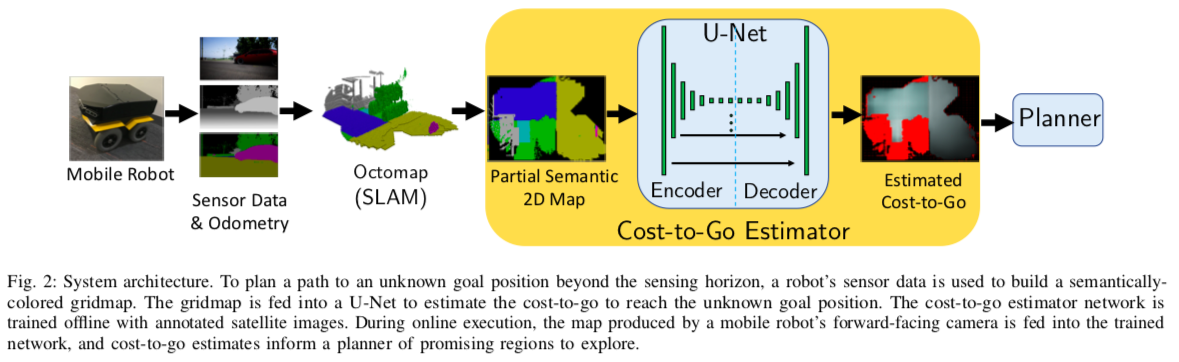
The cost to go
The researchers looked to speed up a robot’s path-planning through a semantic, context-colored world. They developed a new “cost-to-go estimator,” an algorithm that converts a semantic map created by pre-existing SLAM algorithms into a second map, representing the likelihood of any given location being close to the goal.
“This was inspired by image-to-image translation, where you take a picture of a cat and make it look like a dog,” Everett says. “The same type of idea happens here where you take one image that looks like a map of the world, and turn it into this other image that looks like the map of the world but now is colored based on how close different points of the map are to the end goal.”
This cost-to-go map is colorized, in gray-scale, to represent darker regions as locations far from a goal, and lighter regions as areas that are close to the goal. For instance, the sidewalk, coded in yellow in a semantic map, might be translated by the cost-to-go algorithm as a darker region in the new map, compared with a driveway, which is progressively lighter as it approaches the front door — the lightest region in the new map.
The researchers trained this new algorithm on satellite images from Bing Maps containing 77 houses from one urban and three suburban neighborhoods. The system converted a semantic map into a cost-to-go map, and mapped out the most efficient path, following lighter regions in the map, to the end goal. For each satellite image, Everett assigned semantic labels and colors to context features in a typical front yard, such as grey for a front door, blue for a driveway, and green for a hedge.
During this training process, the team also applied masks to each image to mimic the partial view that a robot’s camera would likely have as it traverses a yard.
“Part of the trick to our approach was [giving the system] lots of partial images,” How explains. “So it really had to figure out how all this stuff was interrelated. That’s part of what makes this work robustly.”
The researchers then tested their approach in a simulation of an image of an entirely new house, outside of the training dataset, first using the preexisting SLAM algorithm to generate a semantic map, then applying their new cost-to-go estimator to generate a second map, and path to a goal, in this case, the front door.
The group’s new cost-to-go technique found the front door 189 percent faster than classical navigation algorithms, which do not take context or semantics into account, and instead spend excessive steps exploring areas that are unlikely to be near their goal.
Everett says the results illustrate how robots can use context to efficiently locate a goal, even in unfamiliar, unmapped environments.
“Even if a robot is delivering a package to an environment it’s never been to, there might be clues that will be the same as other places it’s seen,” Everett says. “So the world may be laid out a little differently, but there’s probably some things in common.”
This research is supported, in part, by the Ford Motor Company.
Editor’s Note: This article was republished with permission from MIT News.
The post Semantic SLAM navigation targets last-mile delivery robots appeared first on The Robot Report.