Having worked in industrial automation for most of my career, I’d like to think that I’ve built up a wealth of experience in the field of industrial safety sensors. Familiar with safety laser scanners for over a decade, I have been involved many designs and installations.
I currently work for SICK (UK) Ltd., which invented the safety laser scanner, and I continually see people making the same mistakes time and time again. This short piece highlights, in my opinion, the most common of them.
1. Installation and mounting: Thinking about safety last
If you are going to remember just one point, then this is it. Too many times have I been present at an “almost finished” machine and asked, “Right, where can I stick this scanner?”
Inevitably, what ends up happening is that blind spots (shadows created by obstacles) become apparent all over the place. This requires mechanical “bodges” and maybe even additional scanners to cover the complete area when one scanner may have been sufficient if the cell was designed properly in the first place.
In safety, designing something out is by far the most cost-effective and robust solution. If you know you are going to be using a safety laser scanner, then design it in from the beginning — it could save you a world of pain. Consider blind zones, coverage and the location of hazards.
This also goes for automated guided vehicles (AGVs). For example, the most appropriate position to completely cover an AGV is to have two scanners adjacent to each other on the corners integrated into the vehicle (See Figure 1).
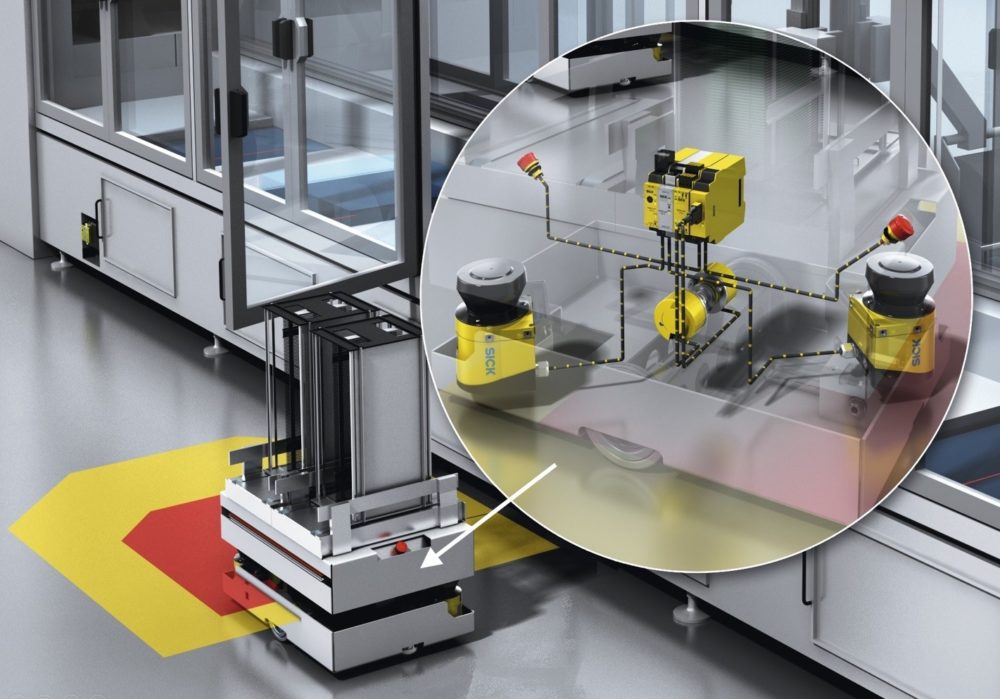
Figure 1: Typical AGV scanner mounting and integration. | Credit: SICK
2. Incorrect multiple sampling values configured
An often misunderstood concept, multiple sampling indicates how often an object has to be scanned in succession before a safety laser scanner reacts. By default and out of the box, this value is usually x2 scans, which is the minimum value. However, this value may range from manufacturer to manufacturer. A higher multiple sampling value reduces the possibility that insects, weld sparks, weather (for outdoor scanners) or other particles cause the machine to shut down.
Increasing the multiple sampling can make it possible to increase a machine’s availability, but it can also have negative effects on the application. Increasing the number of samples is basically adding an OFF-Delay to the system, meaning that your protective field may need to be bigger due to the increase in the total response time.
If a scanner has a robust detection algorithm, then you shouldn’t have to increase this value too much but when this value is changed you could be creating a hazard due to lack of effectiveness of the protective device.
If the value is changed, you should make a note of the safety laser scanner’s new response time and adjust the minimum distance from the hazardous point accordingly to ensure it remains safe.
Furthermore, in vertical applications, if the multiple sampling is set too high, then it may be possible for a person to pass through the protective field without being detected — so care must be taken. For one our latest safety laser scanners, the microScan3, we provide the following advice:
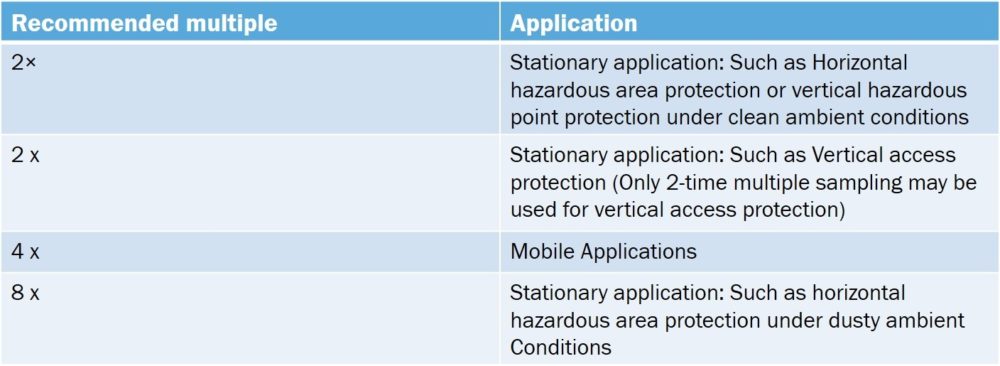
Figure 2: Recommended multiple sampling values. | Credit: SICK
3. Incorrect selection of safety laser scanner
The maximum protective field that a scanner can facilitate is an important feature, but this value alone should not be a deciding factor on whether the scanner is suitable for an application. A safety laser scanner is a Type 3 device, according to IEC 61496, and an Active Opto-Electric Protective Devices responsive to Diffuse Reflection (AOPDDR). This means that it depends on diffuse reflections off of objects. Therefore, to achieve longer ranges, scanners must be more sensitive. In reality, this means that sometimes scanning angle but certainly detection robustness can be sacrificed.
This could lead to a requirement for an increasing number multiple samples and maybe lack of angular resolution. The increased response times and lack of angle could mean that larger protective fields are required and even additional scanners — even though you bought the longer range one. A protective field should be as large as required but as small as possible.
A shorter-range scanner may be more robust than its longer-range big brother and, hence, keep the response time down, reduce the footprint, reduce cost and eliminate annoying false trips.
4. Incorrect resolution selected
The harmonized standard EN ISO 13855 can be used for the positioning of safeguards with respect to the approach speeds of the human body. Persons or parts of the body to be protected may not be recognized or recognized in time if the positioning or configuration is incorrect. The safety laser scanner should be mounted so that crawling beneath, climbing over and standing behind the protective fields is not possible.
If crawling under could create a hazardous situation, then the safety laser scanner should not be mounted any higher than 300 mm. At this height, a resolution of up 70 mm can be selected to ensure that it is possible to detect a human leg. However, it is sometimes not possible to mount the safety laser scanner at this height. If mounted below 300 mm, then a resolution of 50 mm should be used.
It is a very common mistake to mount the scanner lower than 300 mm and leave the resolution on 70mm. Reducing the resolution may also reduce the maximum protective field possible on a safety laser scanner so it is important to check.
5. Ambient/environmental conditions were not considered
Sometimes safety laser scanners just aren’t suitable in an application. Coming from someone who sells and supports these devices, that is a difficult thing to say. However, scanners are electro-sensitive protective equipment and infrared light can be a tricky thing to work with. Scanners have become very robust devices over the last decade with increasingly complex detection techniques (SafeHDDM by SICK) and there are even safety laser scanners certified to work outdoors (outdoorScan3 by SICK).
However, there is a big difference between safety and availability and expectations need to be realistic right from the beginning. A scanner might not maintain 100% machine availability if there is heavy dust, thick steam, excessive wood chippings, or even dandelions constantly in front of the field of view. Even though the scanner will continue to be safe and react to such situations, trips due to ambient conditions may not be acceptable to a user.
For extreme environments, the following question should be asked: “What happens when the scanner is not available due to extreme conditions?” This can be especially true in outdoor application in heavy rain, snow or fog. A full assessment of the ambient conditions and even potentially proof tests should be carried out. This particular issue can become a very difficult, and sometimes impossible, and expensive thing to fix.
6. Non-safe switching of field sets
A field set in a safety laser scanner can consist of multiple different field types. For example, a field set could consist of 4 safe protection fields (Field Set 1) or it could consist of 1 safe protective field, two non-safe warning fields and a safe detection field (Field set 2). See Figure 3.

Figure 3: Safety laser scanner field sets. | Credit: SICK
A scanner can store lots of different fields that can be selected using either hardwired inputs or safe networked inputs (CIP Safety, PROFISAFE, EFI Pro). This is a feature that industry finds very useful for both safety and productivity in Industry 4.0 applications.
However, the safety function (as per EN ISO 13849/EN 62061) for selecting the field set at any particular point in time should normally have the same safety robustness (PL/SIL) as the scanner itself. A safety laser scanner can be used in safety functions up to PLd/SIL2.
If we look at AGVs, for example, usually two rotary encoders are used to switch between fields achieving field switching up to PLe/SIL3. There are now also safety rated rotary encoders that can be used alone to achieve field switching to PLd/SIL2.
However, sometimes the safety of the mode selection is overlooked. For example, if a standard PLC or a single channel limit switch is used for selecting a field set, then this would reduce the PL/SIL of the whole system to possibly PLc or even PLa. An incorrect selection of field set could mean that an AGV is operating with small protective field in combination with a high speed and hence long stopping time, creating a hazardous situation.
Summary
Scanners are complex devices and have been around for a long time with lots of choice in the market with regards to range, connectivity, size and robustness. There are also a lot of variables to consider when designing a safety solution using scanners. If you are new to this technology then it is a good idea to contact the manufacturer for advice on the application of these devices.
Here at SICK we offer complimentary services to our customers such as consultancy, on-site engineering assistance, risk assessment, safety concept and safety verification of electrosensitive protective equipment (ESPEs). We are always happy to answer any questions. If you’d like to get in touch then please do not hesitate.
About the Author
Dr. Martin Kidman is a Functional Safety Engineer and Product Specialist, Machinery Safety at SICK (UK) Ltd. He received his Ph.D. at the University of Liverpool in 2010 and has been involved in industrial automation since 2006 working for various manufacturers of sensors.
Kidman has been at SICK since January 2013 as a product specialist for machinery safety providing services, support and consultancy for industrial safety applications. He is a certified FS Engineer (TUV Rheinland, #13017/16) and regularly delivers seminars and training courses covering functional safety topics. Kidman has also worked for a notified body testing to the Low Voltage Directive in the past.
The post 6 common mistakes when setting up safety laser scanners appeared first on The Robot Report.