The U.S. National Robotics Roadmap was first created 10 years ago. Since then, government agencies, universities, and companies have used it as a reference for where robotics is going. The first roadmap was published in 2009 and then revised in 2013 and 2016. The objective is to publish the fourth version of the roadmap by summer 2020.
The team developing the U.S. National Robotics Roadmap has put out a call to engage about 150 to 200 people from academia and industry to ensure that it is representative of the robotics community’s view of the future. The roadmap will cover manufacturing, service, medical, first-responder, and space robotics.
The revised roadmap will also include considerations related to ethics and workforce. It will cover emerging applications, the key challenges to progress, and what research and development is needed.
Join community workshops
Three one-and-a-half-day workshops will be organized for community input to the roadmap. The workshops will take place as follows:
- Sept. 11-12 in Chicago (organized by Nancy Amato, co-director of the Parasol Lab at Texas A&M University and head of the Department of Computer Science at the University of Ilinois at Urbana-Champaign)
- Oct. 17-18 in Los Angeles (organized by Maja Mataric, Chan Soon-Shiong distinguished professor of computer science, neuroscience, and pediatrics at the University of Southern California)
- Nov. 15-16 in Lowell, Mass. (organized by Holly Yanco, director of the NERVE Center at the University of Massachusetts Lowell)
Participation in these workshops will be by invitation only. To participate, please submit a white paper/position statement of a maximum length of 1.5 pages. What are key use cases for robotics in a five-to-10-year perspective, what are key limitations, and what R&D is needed in that time frame? The white paper can address all three aspects or focus on one of them. The white paper must include the following information:
- Name, affiliation, and e-mail address
- A position statement (1.5 pages max)
Please submit the white paper as regular text or as a PDF file. Statements that are too long will be ignored. Position papers that only focus on current research are not appropriate. A white paper should present a future vision and not merely discuss state of the art.
White papers should be submitted by end of the day Aug. 15, 2019, to roadmapping@robotics-vo.org. Late submissions may not be considered. We will evaluate submitted white papers by Aug. 18 and select people for the workshops by Aug. 19.
Roadmap revision timeline
The workshop reports will be used as the basis for a synthesis of a new roadmap. The nominal timeline is:
- August 2019: Call for white papers
- September – November 2019: Workshops
- December 2019: Workshops reports finalized
- January 2020: Synthesis meeting at UC San Diego
- February 2020: Publish draft roadmap for community feedback
- April 2020: Revision of roadmap based on community feedback
- May 2020: Finalize roadmap with graphics design
- July 2020: Publish roadmap
If you have any questions about the process, the scope, etc., please send e-mail to Henrik I Christensen at hichristensen@eng.ucsd.edu.
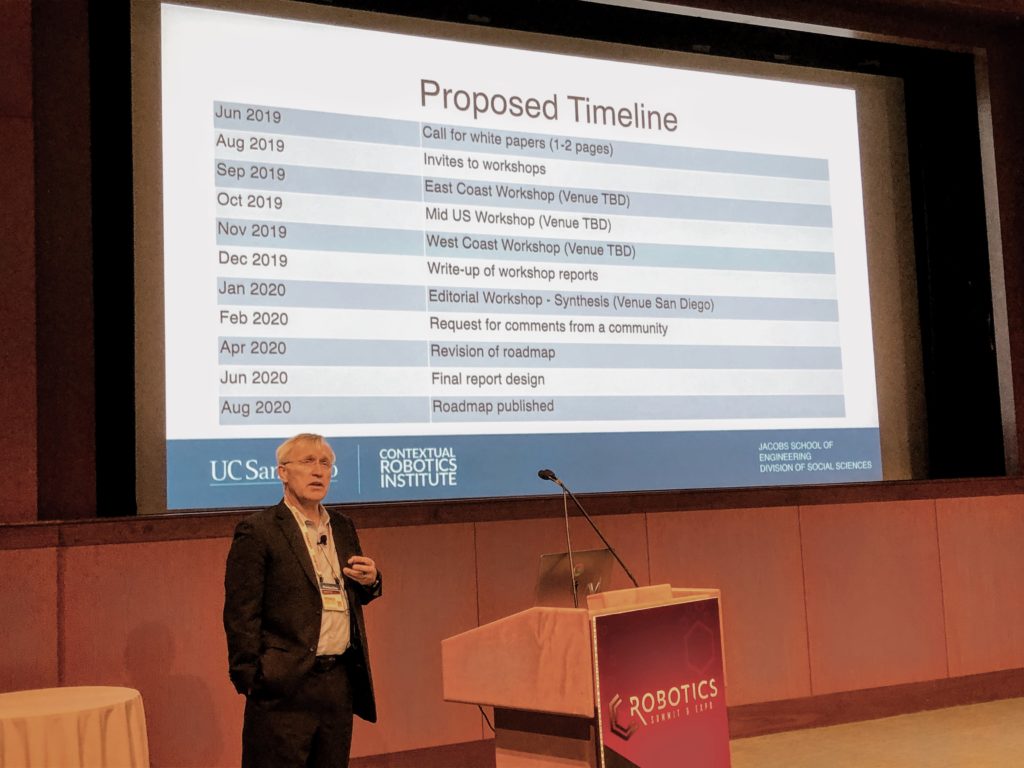
Henrik I Christensen spoke at the Robotics Summit & Expo in Boston.
Editor’s note: Christensen, Qualcomm Chancellor’s Chair of Robot Systems at the University of California San Diego and co-founder of Robust AI, delivered a keynote address at last month’s Robotics Summit & Expo, produced by The Robot Report.
The post U.S. Robotics Roadmap calls for white papers for revision appeared first on The Robot Report.